Unlocking Potential: The Role of Machine Learning Data Labeling in Business Growth
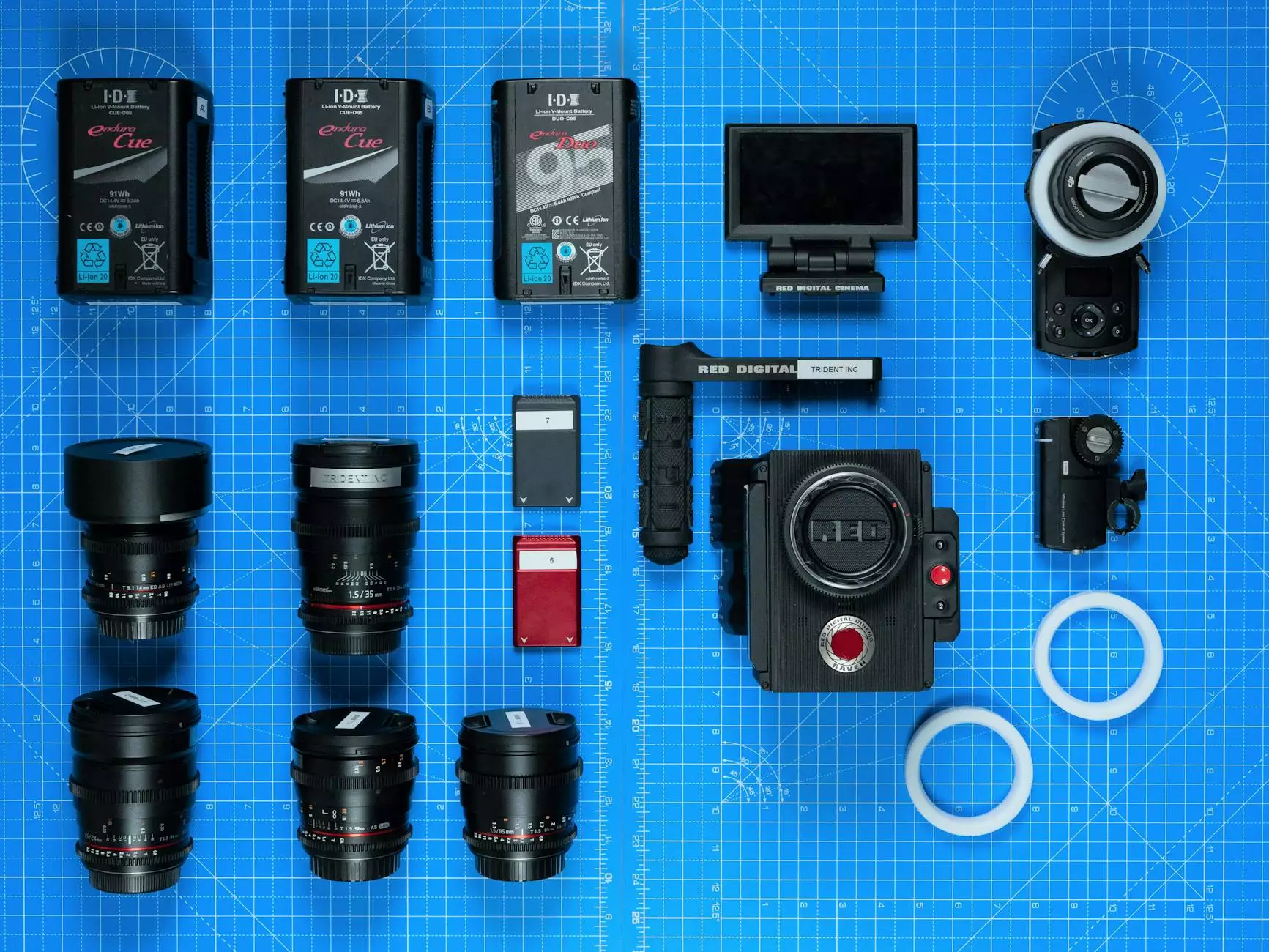
In today's dynamic business environment, leveraging advanced technologies can significantly enhance operational efficiency. One such transformative technology is machine learning data labeling, which plays a crucial role in training algorithms to understand and process data accurately. This article delves into how machine learning data labeling can serve as a powerful tool for businesses, especially in the realms of Home Services and Keys & Locksmiths.
Understanding the Basics of Machine Learning Data Labeling
Machine learning data labeling is the process of annotating data to create training sets for machine learning algorithms. When used correctly, labeled data enables algorithms to learn patterns, make decisions, and improve over time, thereby producing more reliable outputs. The importance of this process cannot be understated, especially when it comes to applications in various business sectors.
Why Machine Learning is Important for Businesses
Embracing machine learning can lead to significant competitive advantages. Here’s how:
- Enhanced Decision Making: Machine learning allows businesses to analyze vast datasets, revealing insights that inform strategic decisions.
- Improved Customer Experience: Personalized recommendations powered by machine learning can enhance customer satisfaction and loyalty.
- Increased Operational Efficiency: Automating tasks through intelligent systems can reduce human error and optimize processes.
- Cost Savings: Businesses can save on labor costs and reduce waste by leveraging automated systems.
The Connection Between Machine Learning Data Labeling and Business Growth
For businesses in sectors like Home Services and Keys & Locksmiths, machine learning data labeling facilitates numerous advancements:
1. Optimizing Customer Interaction
In the service-oriented sector, understanding customer queries and preferences is paramount. By employing machine learning algorithms trained on labeled data, businesses can:
- Analyze customer feedback and interactions.
- Automate responses to FAQs, thereby reducing response time.
- Predict customer needs based on previous interactions.
2. Streamlining Operations
Data labeling aids in creating intelligent systems that optimize resource allocation:
- Predictive maintenance schedules for tools and vehicles.
- Route optimization for service delivery, saving time and costs.
- Inventory management through data-driven demand forecasting.
Key Applications of Machine Learning Data Labeling in Home Services and Locksmiths
Enhancing Security Systems
In the locksmith industry, integrating machine learning data labeling plays a critical role in developing smart security systems. By training models on labeled images of different lock mechanisms, businesses can:
- Identify vulnerabilities in existing security setups.
- Suggest enhanced security measures tailored to customer needs.
Improving Customer Service Through Predictive Analysis
For Home Services businesses, utilizing labeled data for predictive customer service measures can lead to:
- Anticipating service needs based on seasonal trends.
- Automatically scheduling routine maintenance reminders for clients.
A Comprehensive Look at the Data Labeling Process
Implementing an effective machine learning data labeling process involves several critical steps:
Data Collection
The first step is gathering relevant data, which can include:
- Customer service logs.
- Transaction records.
- Service feedback forms.
Data Preprocessing
Once data is collected, it needs to be cleaned and formatted to ensure accuracy. This can involve:
- Removing duplicates and irrelevant entries.
- Standardizing formats across the dataset.
Data Annotation
The core aspect of machine learning data labeling is annotating the cleaned data. This may involve:
- Classifying customer queries into categories.
- Tagging service requests based on urgency and type.
Model Training
Trained models should be evaluated against a portion of unlabelled data to test their accuracy and reliability in real-world scenarios. After multiple iterations, the models can be fine-tuned to improve their predictive power.
Challenges in Machine Learning Data Labeling
While the benefits of machine learning data labeling are immense, there are challenges that businesses may encounter:
- Quality Assurance: Ensuring that labeled data is consistently accurate is critical.
- Scalability: As the volume of data increases, maintaining efficiency in labeling processes can be challenging.
- Hiring Expertise: Finding skilled personnel to accurately label data can be a daunting task.
The Future of Machine Learning Data Labeling in Business
The landscape of data labeling is evolving rapidly. With innovative tools and techniques emerging, the future holds promising prospects:
- Automated Data Labeling: Advancements in AI could reduce the need for extensive human labeling.
- Enhanced Collaboration: Businesses can work collaboratively to share labeled datasets, accelerating the learning process.
Conclusion
The implementation of machine learning data labeling is more than just a technical necessity; it is a strategic asset for businesses, especially in the Home Services and Keys & Locksmiths sectors. By understanding how to collect and label data effectively, organizations can unlock new levels of operational efficiency, enhance customer satisfaction, and ultimately foster growth.
Harnessing the power of machine learning through effective data labeling is not merely a trend; it's an investment into the future of your business.